Ethical Concerns in Machine Learning: Balancing Innovation and Privacy
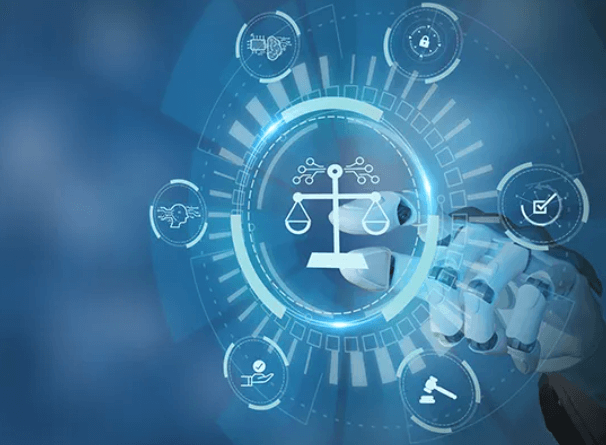
Machine learning (ML) has transformed the way businesses, governments, and individuals use data to make informed decisions. From personalized recommendations to healthcare diagnostics, ML algorithms have unlocked unprecedented levels of innovation. However, with great power comes great responsibility. As machine learning technologies advance, ethical concerns such as privacy, bias, transparency, and accountability have come to the forefront.
In this article, we will explore the key ethical issues associated with machine learning, the impact on individuals and society, and how organizations can balance innovation with responsible use of AI.
1. Introduction to Ethical Concerns in Machine Learning
Machine learning is transforming industries by enabling automation, optimizing decision-making, and uncovering hidden patterns in data. However, the rise of ML also raises significant ethical challenges. As organizations rely more on algorithms to make decisions that affect people’s lives, the ethical implications of these technologies have become more pressing.
From biased hiring algorithms to surveillance technologies, the potential for harm is real. Businesses, regulators, and developers must consider the ethical consequences of their AI systems to ensure that technology is used responsibly and does not infringe on human rights.
See also: Real-World Applications of Machine Learning in Healthcare: Transforming Patient Care
2. The Impact of Machine Learning on Privacy
One of the most critical ethical concerns in machine learning is data privacy. ML models often require large datasets to function effectively, and much of this data is collected from users without explicit consent. Examples of privacy concerns include:
- Data Collection: Companies often collect more data than necessary, leading to potential misuse or breaches.
- Surveillance: Facial recognition systems and location tracking can infringe on personal privacy, especially when used by governments or corporations.
- Informed Consent: Users are often unaware of how their data is being collected, analyzed, or shared.
Balancing Innovation with Privacy:
- Implement data minimization principles to collect only the data needed.
- Use anonymization techniques to protect user identities.
- Provide transparency and clear consent mechanisms for data collection.
3. Bias in Machine Learning Algorithms
Bias is another major ethical issue in machine learning. Algorithms trained on biased data can perpetuate or even amplify existing inequalities, leading to unfair outcomes.
Types of Bias:
- Data Bias: When training data reflects historical inequalities (e.g., racial, gender, or socioeconomic biases).
- Algorithmic Bias: When the design of an ML model introduces unintended biases.
- User Bias: When user interactions influence the behavior of an algorithm, such as in recommendation systems.
Examples:
- Biased hiring algorithms that favor certain demographics.
- Facial recognition systems that misidentify people of color more frequently than others.
- Loan approval systems that discriminate against specific groups.
How to Mitigate Bias:
- Regularly audit ML models for bias.
- Use diverse and representative training datasets.
- Employ fairness-aware machine learning techniques.
4. Lack of Transparency in AI Models
Many machine learning models, especially those based on deep learning, are often referred to as “black boxes” because their internal workings are difficult to understand. This lack of transparency raises ethical concerns, especially when algorithms are used in high-stakes areas like healthcare, criminal justice, or finance.
Issues with Transparency:
- Users and stakeholders may not understand how decisions are made.
- Lack of transparency can lead to mistrust, especially if an algorithm makes a controversial decision.
- Difficulties in auditing AI systems for fairness and accountability.
Solutions:
- Use Explainable AI (XAI) techniques to improve the interpretability of models.
- Provide clear documentation on how ML models work and the data used.
- Ensure that stakeholders are informed about the limitations of AI systems.
5. Accountability and Liability in Machine Learning
As organizations increasingly rely on machine learning algorithms for decision-making, questions about accountability and liability become crucial. If an algorithm makes an incorrect or harmful decision, who is responsible?
Challenges:
- Determining liability in cases where an algorithm’s decision leads to harm (e.g., autonomous vehicles or healthcare diagnostics).
- Holding companies accountable for algorithmic discrimination or errors.
- Ensuring that AI systems comply with ethical and legal standards.
Approaches:
- Establish clear accountability frameworks for AI systems.
- Ensure that developers, companies, and regulators have oversight mechanisms.
- Create guidelines and policies for ethical AI use.
6. The Challenge of Data Security
Machine learning models often require vast amounts of data, which increases the risk of data breaches and cyberattacks. The storage, processing, and sharing of sensitive data need to be handled with the highest security standards.
Security Concerns:
- Data Breaches: Unauthorized access to sensitive information.
- Model Attacks: Techniques like adversarial attacks can manipulate ML models to produce incorrect outputs.
- Intellectual Property Theft: Competitors or malicious actors may steal proprietary ML models.
Best Practices for Data Security:
- Encrypt sensitive data both at rest and in transit.
- Implement robust authentication and access controls.
- Conduct regular security audits of ML systems.
7. The Role of Fairness in AI Decision-Making
Ensuring fairness in AI is essential for building trust and avoiding discrimination. Machine learning systems that influence access to loans, jobs, or healthcare must be designed to treat all individuals equitably.
Key Considerations:
- Define what fairness means for your specific application (e.g., equal opportunity, demographic parity).
- Use fairness metrics to evaluate and adjust ML models.
- Involve diverse stakeholders in the design and deployment of AI systems.
8. Balancing Innovation with Ethical AI Practices
While machine learning offers vast potential for innovation, it must be balanced with ethical considerations. Businesses that prioritize ethical AI practices are likely to gain trust and loyalty from customers, regulators, and society.
Strategies for Ethical AI:
- Ethical Guidelines: Establish clear ethical principles for AI development.
- Diverse Teams: Involve diverse perspectives to identify potential ethical issues.
- Ongoing Training: Educate employees about the ethical implications of AI.
9. How to Address Ethical Concerns in Machine Learning
Here are some actionable steps organizations can take to address ethical concerns:
- Conduct Ethical Audits: Regularly review your AI systems for potential ethical issues.
- Adopt Responsible AI Frameworks: Leverage frameworks like the AI Ethics Guidelines from organizations like the EU and IEEE.
- Engage with Regulators: Stay updated on emerging AI regulations to ensure compliance.
- Focus on Explainability: Make AI systems transparent to stakeholders to build trust.
10. Frequently Asked Questions (FAQs)
Q1. What are the main ethical issues in machine learning?
The key issues include privacy, bias, transparency, accountability, and data security.
Q2. How can companies prevent bias in their machine learning models?
By using diverse datasets, regularly auditing algorithms, and involving diverse teams in the development process.
Q3. What is Explainable AI, and why is it important?
Explainable AI (XAI) aims to make AI systems more transparent, helping users understand how decisions are made and ensuring accountability.
Q4. Are there regulations governing the ethical use of AI?
Yes, several countries and organizations are developing AI regulations, including the EU’s AI Act and guidelines from the OECD.
Q5. How can businesses balance innovation with ethical AI?
By adopting responsible AI practices, engaging with stakeholders, and prioritizing transparency and fairness.
Conclusion
Machine learning is driving unprecedented levels of innovation, but it also raises complex ethical concerns that cannot be ignored. As organizations continue to integrate ML into their operations, striking a balance between innovation and ethical responsibility is essential. By addressing privacy, bias, transparency, and accountability, businesses can build AI systems that are not only powerful but also fair and trustworthy.