Real-World Applications of Machine Learning in Healthcare: Transforming Patient Care
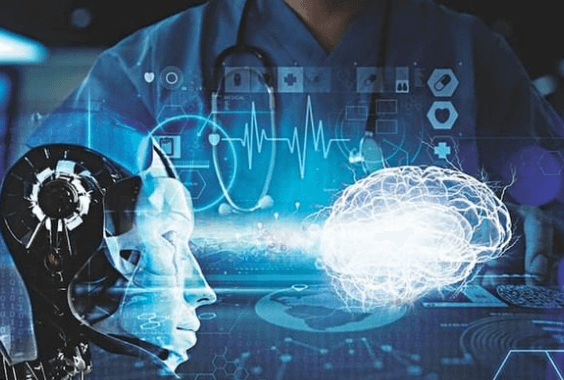
The healthcare industry is undergoing a significant transformation with the integration of machine learning (ML) technologies. These advancements are not only enhancing patient care but also improving operational efficiency and reducing costs. From early disease detection to personalized medicine, machine learning is playing a pivotal role in the healthcare sector.
This article delves into real-world applications of machine learning in healthcare, highlighting how it’s reshaping patient care and empowering healthcare professionals.
1. Introduction to Machine Learning in Healthcare
Machine learning is a branch of artificial intelligence (AI) that uses algorithms to analyze data, identify patterns, and make predictions. In healthcare, machine learning can analyze vast amounts of medical data, helping healthcare professionals make informed decisions that improve patient outcomes. The technology is revolutionizing diagnostics, treatment plans, and patient care management by enabling faster and more accurate decision-making.
See also: Understanding Neural Networks: The Foundation of Deep Learning
2. How Machine Learning Works in the Healthcare Sector
Machine learning models are trained using historical medical data, such as electronic health records (EHRs), medical imaging, lab results, and patient feedback. By learning from this data, the models can:
- Identify patterns that may not be visible to humans.
- Make predictions about disease progression.
- Suggest personalized treatment plans.
- Detect anomalies in real-time patient monitoring.
The more data the system processes, the better it becomes at making accurate predictions and recommendations.
3. Benefits of Machine Learning in Healthcare
- Improved Diagnostics: ML algorithms can detect diseases earlier and with higher accuracy.
- Personalized Treatments: Tailored therapies based on a patient’s genetic makeup and medical history.
- Operational Efficiency: Automating administrative tasks, reducing paperwork, and optimizing resource allocation.
- Cost Reduction: Reducing hospital readmissions, optimizing treatment plans, and lowering overall healthcare costs.
- Predictive Analytics: Anticipating outbreaks, managing patient flow, and optimizing hospital operations.
4. Real-World Applications of Machine Learning in Healthcare
Early Disease Detection and Diagnostics
Machine learning algorithms are transforming the way diseases are detected and diagnosed. For example:
- Cancer Detection: ML models can analyze mammograms, CT scans, and MRIs to detect cancerous lesions with higher accuracy than human radiologists. This early detection can significantly improve survival rates.
- Diabetes Prediction: Predictive models can identify patients at risk of developing diabetes based on historical health data, enabling early interventions.
- Cardiovascular Disease: Algorithms can analyze ECGs, blood pressure, and cholesterol levels to predict heart attacks or strokes before they occur.
Personalized Treatment Plans
Personalized medicine involves tailoring treatments based on an individual’s genetic profile, lifestyle, and medical history. Machine learning enables:
- Genomic Analysis: Analyzing genetic data to identify mutations and recommend targeted therapies, especially in cancer treatment.
- Treatment Recommendations: Machine learning models can predict how patients will respond to specific medications, optimizing drug dosages and reducing side effects.
Drug Discovery and Development
The process of developing new drugs is long and expensive, but machine learning can accelerate this process:
- Drug Repurposing: AI can analyze existing drugs and find new therapeutic uses, reducing the time and cost of development.
- Clinical Trials: ML algorithms can identify suitable candidates for clinical trials, predict patient responses, and improve trial success rates.
Predictive Analytics for Patient Outcomes
Predictive analytics leverages historical data to forecast future health outcomes:
- Hospital Readmission: Identifying patients at high risk of readmission and intervening early to prevent it.
- Disease Progression: Tracking chronic conditions like Alzheimer’s or Parkinson’s to predict disease progression and adjust treatment plans accordingly.
- Risk Scoring: Predicting patient complications, such as infections or post-surgery issues, to proactively manage care.
Medical Imaging Analysis
Machine learning is revolutionizing the analysis of medical images by:
- Image Segmentation: Identifying tumors, lesions, and other abnormalities in X-rays, MRIs, and CT scans.
- Automated Diagnostics: Reducing diagnostic errors and speeding up the time it takes to interpret medical images, leading to quicker treatment decisions.
Remote Patient Monitoring and Telehealth
The rise of remote healthcare has been accelerated by the pandemic, and machine learning plays a crucial role:
- Wearable Devices: Analyzing data from wearables like smartwatches to monitor heart rate, glucose levels, and sleep patterns in real time.
- Telehealth Platforms: AI-powered chatbots and virtual assistants help triage patients, provide initial consultations, and suggest follow-up actions.
5. Challenges of Implementing Machine Learning in Healthcare
Despite its benefits, there are challenges in adopting machine learning in healthcare:
- Data Privacy: Protecting sensitive patient data from breaches.
- Integration: Integrating ML systems with existing healthcare IT infrastructure.
- Bias in Algorithms: Ensuring that ML models are unbiased and fair, especially when trained on historical data that may contain biases.
- Regulatory Compliance: Navigating strict regulations in healthcare, such as HIPAA, to ensure compliance.
6. The Role of Ethics and Data Privacy in Healthcare AI
The use of machine learning in healthcare must adhere to strict ethical standards:
- Patient Consent: Ensuring patients are informed about how their data will be used.
- Bias Mitigation: Developing algorithms that are transparent, unbiased, and inclusive.
- Data Security: Using encryption and secure data storage to protect patient information.
7. Future Trends in Machine Learning for Healthcare
The future of machine learning in healthcare looks promising, with trends such as:
- Explainable AI: Making ML models more interpretable to build trust among healthcare professionals.
- Federated Learning: Training algorithms on decentralized data sources without compromising privacy.
- Precision Medicine: Leveraging big data and ML for highly targeted therapies.
8. How to Get Started with Machine Learning in Healthcare
For healthcare professionals or organizations interested in adopting ML, consider:
- Education: Take courses in AI, data science, and healthcare analytics.
- Collaborate with Experts: Partner with data scientists and technology companies.
- Leverage Open-Source Tools: Use platforms like TensorFlow, PyTorch, and Scikit-learn to build and deploy ML models.
- Focus on Data Security: Implement robust data protection measures to comply with regulations.
9. Conclusion: The Impact of Machine Learning on Healthcare’s Future
Machine learning is reshaping the healthcare industry by making diagnostics more accurate, treatments more personalized, and healthcare systems more efficient. As ML continues to advance, it holds the potential to revolutionize patient care, reduce healthcare costs, and improve overall health outcomes. However, the successful integration of machine learning in healthcare will require addressing ethical concerns, ensuring data privacy, and building trust among patients and healthcare providers.
10. Frequently Asked Questions (FAQs)
Q1. How is machine learning used in diagnostics?
ML algorithms analyze medical data such as imaging scans, lab results, and genetic information to diagnose diseases early and accurately.
Q2. Can machine learning improve patient outcomes?
Yes, by predicting disease progression, personalizing treatments, and enabling real-time patient monitoring, ML improves patient care and outcomes.
Q3. What are the challenges of using machine learning in healthcare?
Challenges include data privacy, algorithm bias, integration with existing systems, and regulatory compliance.
Q4. How does machine learning help in drug discovery?
ML accelerates the drug discovery process by identifying potential drug compounds, predicting their effects, and repurposing existing drugs for new uses.
Q5. Are there ethical concerns with using AI in healthcare?
Yes, concerns include patient consent, data privacy, algorithm transparency, and ensuring that AI systems do not perpetuate biases in healthcare.
Q6. How can hospitals start implementing machine learning?
Hospitals can begin by investing in data infrastructure, collaborating with AI experts, and focusing on specific areas like diagnostics or patient monitoring to start leveraging ML.