The Role of Machine Learning in Predictive Analytics: Unlocking the Future of Data-Driven Decisions
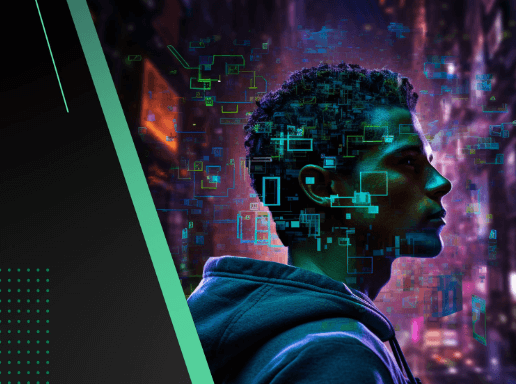
In today’s competitive landscape, data has become the backbone of successful business strategies. Companies are leveraging predictive analytics to anticipate future trends, optimize operations, and gain a competitive edge. At the heart of these efforts is machine learning (ML), a powerful tool that enhances the accuracy, speed, and efficiency of predictive analytics.
This article explores the role of machine learning in predictive analytics, the benefits it brings to various industries, and how businesses can leverage ML to stay ahead of the curve.
1. Introduction to Predictive Analytics
Predictive analytics involves using historical data, statistical algorithms, and machine learning techniques to predict future outcomes. Businesses use predictive analytics to make better decisions, improve efficiency, and stay competitive in their industries.
From forecasting sales trends to identifying potential risks, predictive analytics helps organizations move from reactive to proactive decision-making. In recent years, machine learning has become a game-changer in this field by significantly enhancing the speed and accuracy of predictions.
See also: Real-World Applications of Machine Learning in Healthcare: Transforming Patient Care
2. What is Machine Learning and How Does It Fit into Predictive Analytics?
Machine learning is a branch of artificial intelligence (AI) that focuses on training models to learn from data and make predictions or decisions with minimal human intervention. In predictive analytics, ML algorithms analyze historical data to identify patterns and forecast future outcomes.
Unlike traditional predictive models that rely heavily on human-defined rules, machine learning models automatically adjust and improve over time as they are exposed to more data. This makes them particularly effective in handling complex, large datasets where traditional approaches might fall short.
3. Benefits of Using Machine Learning in Predictive Analytics
- Increased Accuracy: ML algorithms can process vast amounts of data quickly, identifying patterns and trends that might be missed by traditional models.
- Scalability: Machine learning models can handle large and complex datasets, making them ideal for businesses with growing data needs.
- Real-Time Predictions: By leveraging real-time data, ML models can provide up-to-date forecasts, enabling businesses to make faster decisions.
- Automation: Machine learning reduces the need for manual intervention, freeing up resources and allowing teams to focus on strategy rather than data processing.
- Continuous Improvement: ML models learn and improve over time, ensuring predictions become more accurate as more data is collected.
4. How Machine Learning Models Enhance Predictive Accuracy
Machine learning models enhance predictive analytics by using techniques such as:
- Regression Analysis: For predicting continuous outcomes like sales growth or stock prices.
- Classification Models: Used to categorize data into predefined classes, such as identifying potential customer churn.
- Time Series Forecasting: Helps predict trends over time, making it ideal for demand planning and resource allocation.
- Clustering Algorithms: Group similar data points together, which can be used for customer segmentation and market analysis.
- Anomaly Detection: Identifies unusual patterns that may indicate fraud, equipment failure, or other critical issues.
5. Key Applications of Machine Learning in Predictive Analytics
Sales and Revenue Forecasting
Machine learning algorithms analyze past sales data, market conditions, and consumer behavior to predict future sales trends. This helps businesses optimize inventory, manage resources, and plan marketing strategies.
Example: Retailers use ML to predict holiday sales spikes, ensuring they have enough stock on hand to meet demand.
Customer Churn Prediction
Retaining customers is more cost-effective than acquiring new ones. ML models can identify customers who are likely to leave by analyzing their behavior patterns, enabling companies to take proactive measures.
Example: Subscription-based services use predictive analytics to offer personalized discounts or incentives to retain customers at risk of churning.
Supply Chain Optimization
ML-powered predictive analytics helps companies optimize their supply chains by forecasting demand, identifying bottlenecks, and improving delivery times.
Example: Logistics companies use predictive analytics to optimize shipping routes, reduce fuel costs, and improve delivery efficiency.
Financial Risk Management
Machine learning models can analyze historical financial data to predict potential risks, such as loan defaults or stock market fluctuations. This helps financial institutions make better lending decisions and mitigate risk.
Example: Banks use ML algorithms to assess the creditworthiness of loan applicants based on past behavior and current market conditions.
Healthcare and Patient Outcomes
Predictive analytics in healthcare uses ML to predict patient outcomes, personalize treatments, and identify potential health risks early on.
Example: Hospitals use ML models to predict patient readmissions, enabling them to implement preventive care measures and improve patient outcomes.
6. Challenges of Implementing Machine Learning in Predictive Analytics
Despite its benefits, using machine learning in predictive analytics comes with challenges:
- Data Quality: ML models require high-quality, clean data for accurate predictions. Incomplete or biased data can lead to incorrect forecasts.
- Integration: Integrating ML models into existing systems can be complex and time-consuming.
- Interpretability: Some ML models, especially deep learning models, act as “black boxes,” making it difficult to understand how predictions are made.
- Skill Gap: Implementing machine learning requires specialized skills in data science, which may be a barrier for smaller businesses.
7. The Future of Predictive Analytics with Machine Learning
As machine learning technology continues to evolve, we can expect to see more automated predictive analytics tools that require less manual intervention. Future trends include:
- Explainable AI (XAI): Making machine learning models more transparent and interpretable.
- AutoML: Tools that automate the process of building machine learning models, making predictive analytics more accessible to non-experts.
- Real-Time Analytics: Leveraging ML models to make real-time predictions, especially in industries like finance, e-commerce, and healthcare.
8. How to Get Started with Machine Learning in Predictive Analytics
For businesses looking to implement machine learning in their predictive analytics strategy:
- Assess Your Data: Ensure you have access to clean, high-quality data.
- Choose the Right Tools: Use platforms like Python (Scikit-learn, TensorFlow), R, or cloud-based services like AWS Machine Learning and Azure AI.
- Start Small: Begin with a specific use case, such as customer churn prediction or sales forecasting, before scaling up.
- Invest in Training: Equip your team with the necessary skills through courses in data science, machine learning, and predictive analytics.
9. Frequently Asked Questions (FAQs)
Q1. What is the difference between predictive analytics and machine learning?
Predictive analytics focuses on forecasting future outcomes based on historical data, while machine learning uses algorithms to improve the accuracy of these predictions over time.
Q2. Can small businesses benefit from machine learning in predictive analytics?
Yes, with the availability of user-friendly tools and cloud-based platforms, even small businesses can leverage machine learning for predictive insights.
Q3. How long does it take to implement machine learning for predictive analytics?
The timeline depends on the complexity of the project, but most implementations can take a few weeks to several months.
Q4. What industries benefit most from predictive analytics?
Industries like retail, finance, healthcare, logistics, and manufacturing gain significant value from predictive analytics.
Q5. What are the best tools for implementing machine learning in predictive analytics?
Popular tools include Python, R, Power BI, and cloud platforms like AWS SageMaker and Google AI.
Conclusion
The integration of machine learning into predictive analytics is transforming how businesses operate, enabling them to make proactive, data-driven decisions. By leveraging the power of ML, organizations can gain deeper insights, optimize processes, and remain agile in an ever-changing market.